How Machine Learning Helps Improve Cybersecurity
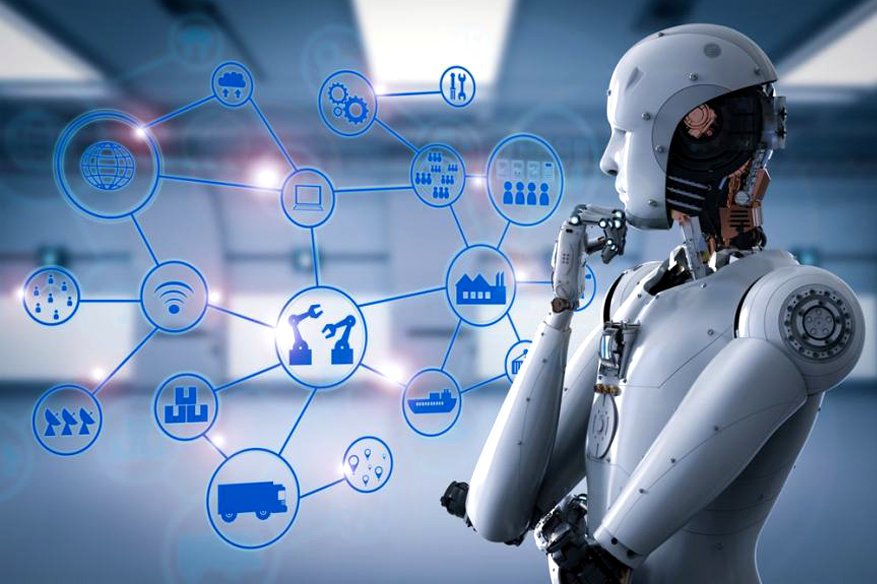
Cyberattacks have increased on an unprecedented scale. Reasons are many. The main reason obviously is our increasing dependence on computing devices (computers, smartphones etc) and the internet for our day-to-day needs. It’s today a world of quickly evolving technologies. The technology that we depend on today has interconnectedness as one of its salient features. This, plus our habit of using unsecured networks and devices (like, for example, public Wi-Fi) for convenience’s sake, too has proven to be the cause for an unprecedented increase in cyberattacks.
Of the various technologies that we use today to prevent cyberattacks and to ensure cybersecurity, machine learning deserves special mention. Machine learning definitely is a great technology that offers some highly efficient security solutions and thus helps prevent cybercrime.
Today, we discuss how machine learning helps improve and ensure cybersecurity in today’s world…
Today, we have many machine learning apps that are used for enhancing cybersecurity. There are many such apps that help monitor networks for cybersecurity issues and to detect vulnerabilities or breaches. Such apps also help enterprises generate automated responses whenever there are cyberattacks. Let’s take a look at how these apps work and how they can be used for security purposes like spam detection, risk detection, detection of phishing attacks and malware detection.
Machine learning apps and spam detection
Machine learning apps play a very important role when it comes to performing spam detection. Different reports suggest that more than half of all email today is spam, and hence there’s an increasing need for spam filters which could effectively block such spam from reaching inboxes and causing trouble. It’s among such spam that malware-laden phishing emails too feature. Today we have robust machine learning-powered spam filters, which work based on different sets of rules to identify and filter spam and which are also cost-effective. That these machine learning-powered spam filters are highly flexible and efficient compared to other knowledge-based methods makes them more suited for combating cybercrime in today’s context. Such machine-learning spam filtering tools work based on entirely dynamic kinds of algorithms, which are based on pre-classified datasets that classify emails as spam or not spam based on many features, including the hyperlinks, the attachments, the word frequency count, the HTML tags, the length of the email, the IP address etc.
Machine learning apps and risk detection
Risk detection and responding to potential risks on a timely basis are all part of the very foundations of cybersecurity. Machine learning apps that are used for cybersecurity help monitor, analyze and respond to all kinds of threats and attacks that happen on the networks, the software and the applications, plus the hardware as well. It has to be remembered that infiltration or infection of a network happens much before detection; attackers could infiltrate systems or networks and remain there without doing anything for many months before launching an attack. It’s here that machine learning comes in handy. Machine learning plays a key role in identifying and detecting cybercrime, in protecting networks and their components from all kinds of risk, and in response and recovery as well.
Detection of phishing attacks using machine learning
Most research data show that cybercriminals are increasingly using phishing techniques to launch cyberattacks. In fact, phishing techniques are the most popular among all techniques used to launch attacks. All internet users get phishing emails delivered to their inboxes on a regular basis and hence detection of phishing attacks is important as regards preventing cybercrime. Phishing attacks could lead to the breach of sensitive personal data including credit card data, banking data, login credentials, intellectual property etc. Phishing attacks are widely used for launching ransomware strikes as well. There are different kinds of phishing attacks and hence anti-phishing methods basically fall under three main groups, namely detective methods, preventive methods and corrective methods. Machine learning algorithms are widely used to help detect phishing emails or websites. This is done by monitoring and analyzing data and related features like the number of links, IP addresses, IP-based URLs, JavaScript presence etc.
Machine learning and malware detection
For long we have had traditional malware detection methods which focused on identifying features like hashes, file properties, code fragments etc. But with the introduction of server-side polymorphism, such detection methods have become irrelevant and obsolete. Today, we have worked out a big shift from the former rule-based malware-detection methods and focus more on detecting malware by analyzing files during the pre-execution phase itself using machine learning. Detecting advanced malware attacks, including ransomware attacks, have thus become easier and more effective, thanks to machine learning. We also use deep learning algorithms to detect rare, high-profile targeted attacks. Thus, machine learning is helping us detect all kinds of malware including trojans, ransomware, adware, spyware etc.
Machine learning has its own limitations as well!
Machine learning, which has immense possibilities when it comes to preventing cybercrime, has its limitations as well. For example, there are ambiguities relating to the definitions of activities as ‘normal’ or ‘anomalous’. There are also issues pertaining to adaptability to new patterns and drastically changing methods of cyberattacks. Fake positives also pose a headache to machine learning methods.
Well, despite these limitations, it’s to be mentioned that machine learning is definitely helping us in ensuring improved cybersecurity. The limitations would in due course be overcome, and machine learning would definitely offer us more possibilities as regards cybercrime detection and prevention.
Related Blogs:
Man Vs. Machines: Employing Artificial Intelligence in Cybersecurity